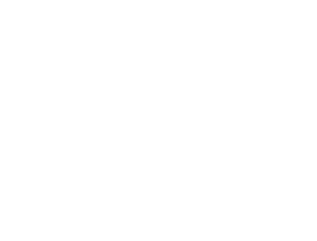
Characterization
Accurate noise mitigation requires one to fully understand what types of noise are present in systems. The characterization thrust will develop quantum characterization methods with an emphasis on robust, scalable, and platform independent methods that can be incorporated into automated routines.
Efficiency methods for multi-qubit Quantum Noise Spectroscopy
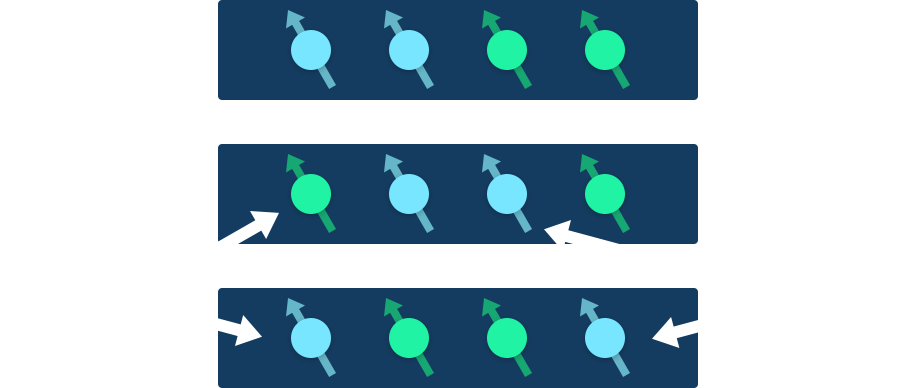
Scalable methods for quantum noise spectroscopy though encoded spaces
JHU/APL and Dartmouth
Bayesian Parameter Learning
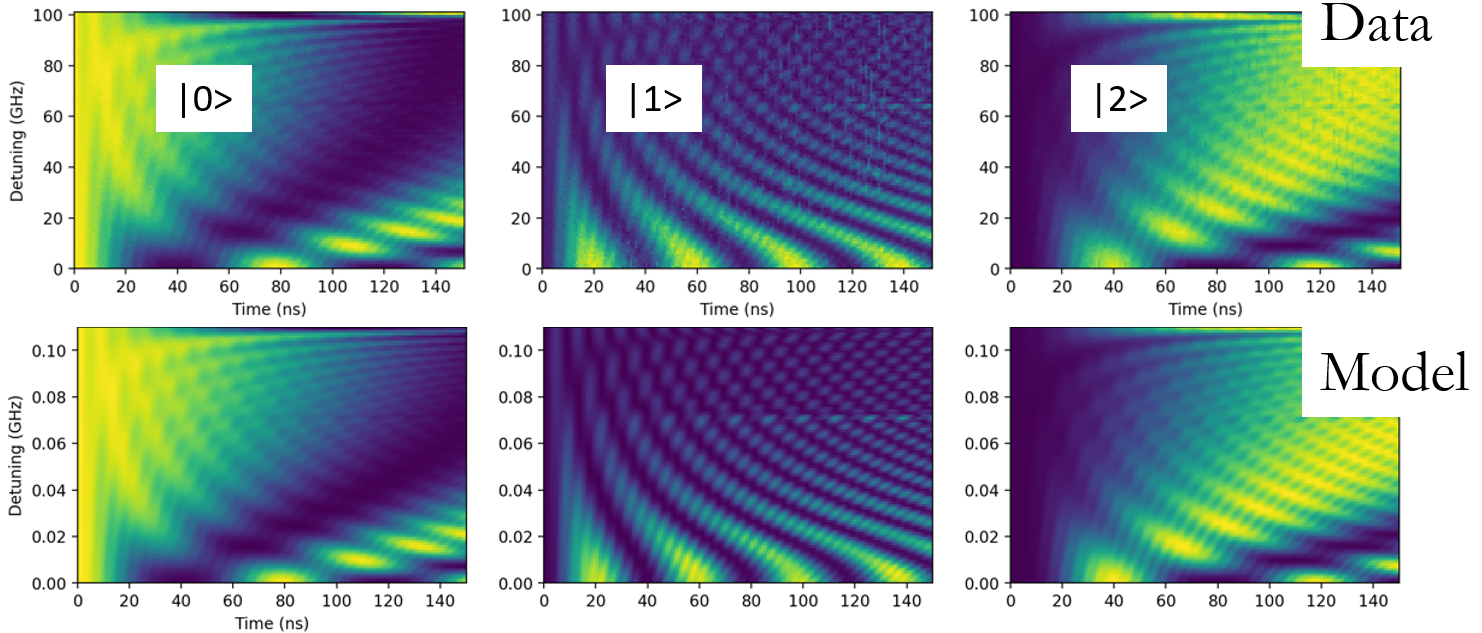
Parameterized models together with Bayesian experimental design allow one to rapidly infer model
Lawrence Livermore National Lab
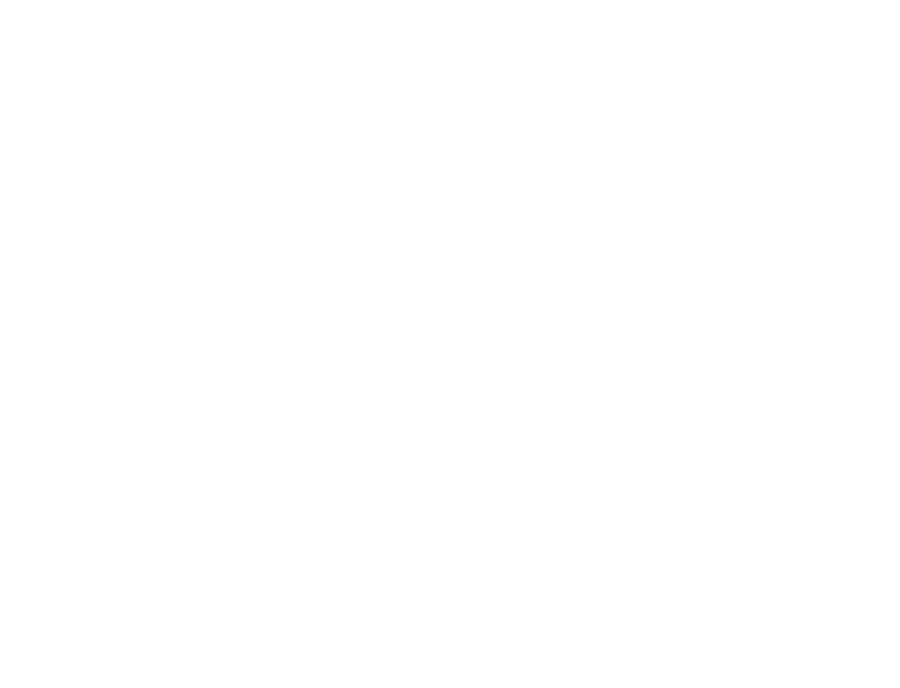
Gate-based Control
This thrust will develop quantum control methods at the circuit or gate level to improve error-resilience and robustness for near-term quantum algorithms. Emphasis will be places on hardware-agnostic general methods that can be used across platforms.
Algorithmic Error Mitigation
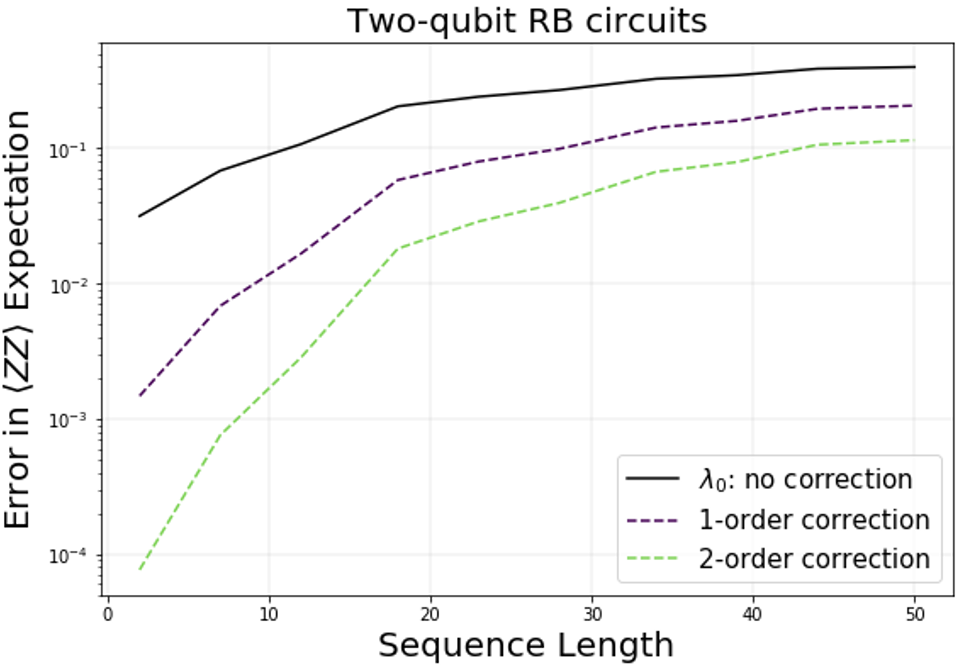
Addition of realistic noise into zero-noise extrapolation for improved performance
Unitary Fund
Pulse-Level Error Mitigation
Reinforcement
Learning
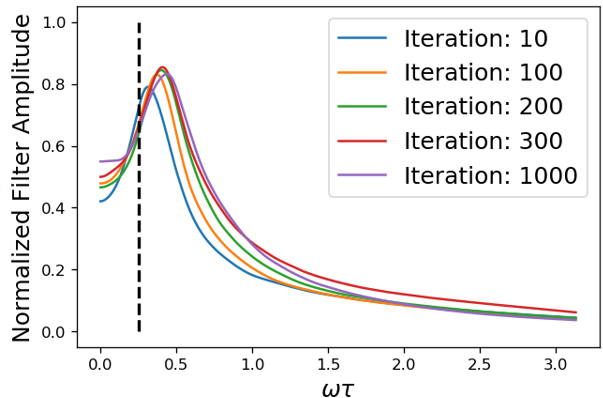
Filter shaping through DRL for rapid gate synthesis
JHU/APL and Darthmouth
Circuit-Level Error Mitigation
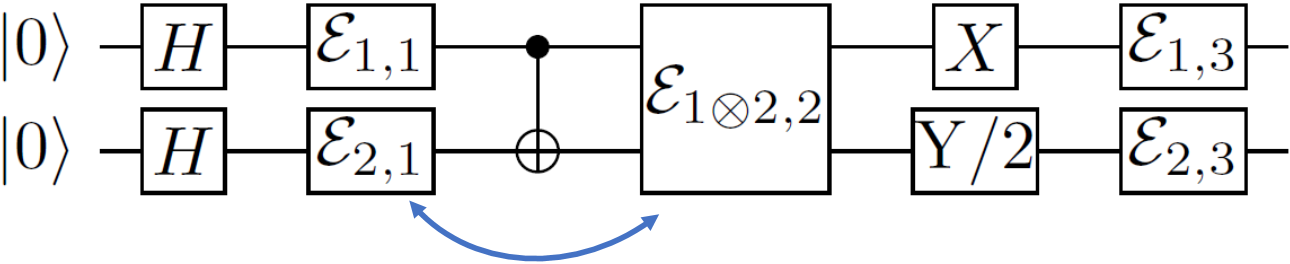
Correlations maintained in time through circuit level error model
JHU/APL and Darthmouth
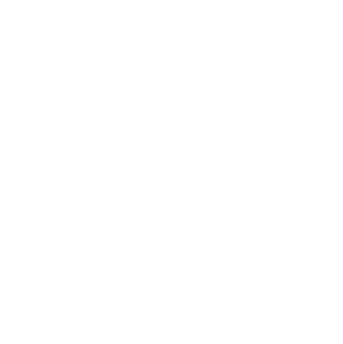
Aggregated Control
This thrust will focus on developing optimal control methods for directly synthesizing large unitary operations into aggregated instructions to reduce circuit depth. This will enable fast, low-noise operation on NISQ quantum processors.
Aggregated Control
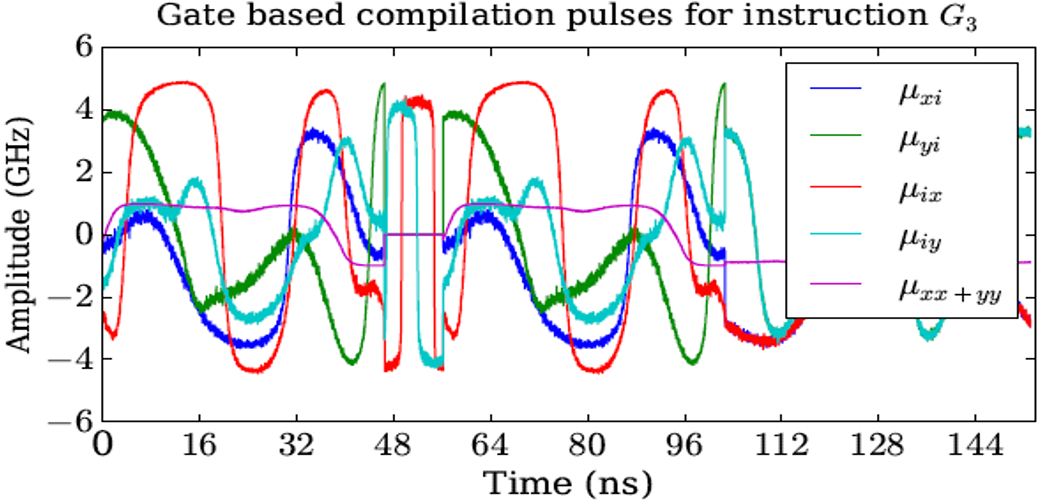
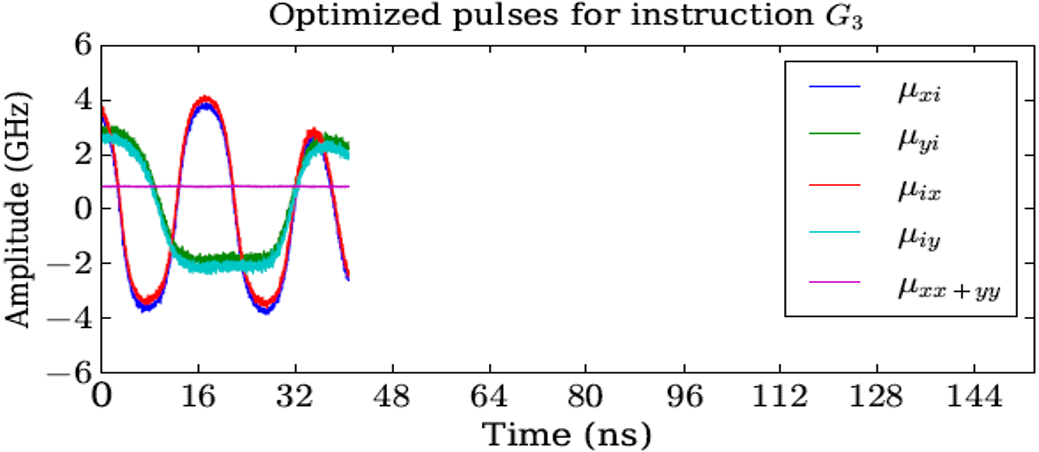
Multiple controls (top) for a circuit are aggregated into a much shorter set of controls (bottom) for improved noise resilience
Chicago and Lawrence Livermore National Lab
HPC Based Control Optimization & Scaling
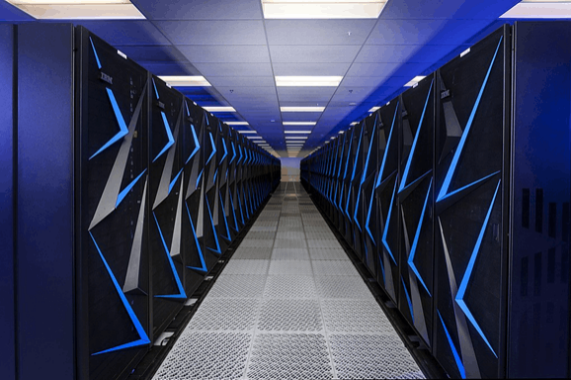
- 20 qubits: 1.1 trillion DOF
- Largest seismic simulation on 1200 nodes of summit: 2.5 trillion DOF + 126,000 timesteps
- Large HPC-based routines should allow for multi-qubit control optimizations
Chicago and Lawrence Livermore National Lab